“The goal of a test is not to get a lift, but rather to get a learning.” – Dr. Flint McGlaughlin, Managing Director and CEO, MECLABS
Many marketers have latched onto A/B testing as a way to improve marketing results. And, they can certainly do that. However, to really drive sustainable returns, you must look past a test that simply tells you to use the red button instead of the blue button, and instead see what split testing is teaching you about your customers.
At MECLABS, we call this “Customer Theory.”
Knowing enough to predict
The Customer Theory is an understanding of the customer that enables us to more accurately predict the total response to a given offer.
In an era of big data, it can be overwhelming to manage results, metrics and numbers. Our understanding of Customer Theory focuses solely on the information that teaches marketers about the customer decision-making process, allowing us to more accurately predict buyer behavior, without being bogged down by superfluous data.
Let me give you an insider’s look at how we do that here at MECLABS. In a previous PPC ad experiment with a MECLABS Research Partner, our researchers ran the following four treatments. Treatment #4 was the winner.
At a very basic level, they learned a more effective PPC ad from this split testing. But, our researchers didn’t stop there. They asked a more fundamental question – what can we predict based on these results? – and ran a follow-up experiment to test their hypothesis.
EXPERIMENT BACKGROUND
Experiment ID: Research Partner Content Approach
Location: MarketingExperiments Research Library
Test Protocol Number: TP4068
Research Notes:
Background: Medical provider specializing in treating chronic back pain. They are the sole providers of a minimally invasive, innovative pain management procedure.
Goal: To plan a content marketing strategy based on which approach generates more appeal in condition-based searchers.
Primary Research Question: Which content approach will achieve a higher clickthrough rate?
Approach: A/B Multifactor Split Test
Our team uses the MECLABS Test Protocol to record the hypothesis, research question and variables, and to ensure that we learn from all of our tests. Here’s a peek at some information from the Test Protocol for this experiment:
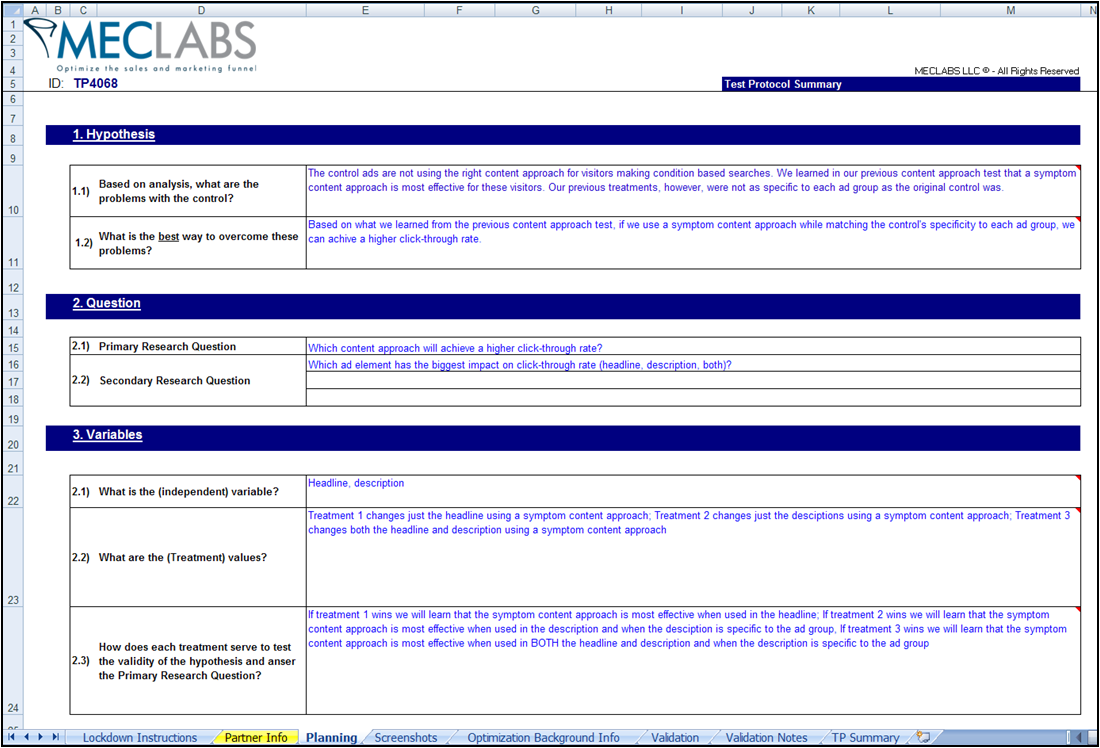
CONTROL
Here is a look at the control ad group:
Here is the general hypothesis from the Test Protocol:
“Based on what we learned from the previous content approach test, if we use a symptom content approach while matching the control’s specificity to each ad group, we can achieve a higher clickthrough rate.”
TREATMENT #1
How treatment #1 will test the hypothesis, according to the Test Protocol:
“If treatment 1 wins, we will learn that the symptom content approach is most effective only when used in the headline.”
TREATMENT #2
How treatment #2 will test the hypothesis, according to the Test Protocol:
“If treatment 2 wins, we will learn that the symptom content approach is most effective when used in the description and when the description is specific to the ad group.”
TREATMENT #3
Here is how treatment #3 will test the hypothesis, according to the Test Protocol:
“If treatment 3 wins, we will learn that the symptom content approach is most effective when used in BOTH the headline and description, and when the description is specific to the ad group.”
RESULTS
What You Need to Understand: By applying the insight from the previous test and inserting ‘symptoms’ into both the headline and description, the team was able to create more successful treatments across all ad groups.
For more information about this experiment, the previous experiment that helped inform this experiment, and customer theory, you can watch the full, free video replay of the Web clinic, “What Your Customers Want: How to predict customer behavior for maximum ROI.”
Related Resources:
A/B Testing: Think like the customer
Marketing Optimization: How your peers predict customer behavior
I saw the web clinic on this, and I’m curious how you were able to split test entire ad groups. Were they set up as separate adgroups? Or were all of the ads set to evenly rotate in one adgroup, and you just split up the ads’ data to get the results for each treatment?
Hi Joe,
I spoke with one of our researchers about your question and a quick summary of our conversation was that the control ads were running in separate ad groups for each of the back pain conditions they were testing in.
Each treatment was run in the respective ad group with the control ad it was testing against and was set to rotate evenly with that control ad.
Thanks for the great question!
@John Tackett
John,
Thank you for the answer, that makes much more sense. I guess I was just a little confused by the wording in the post. I appreciate the response.